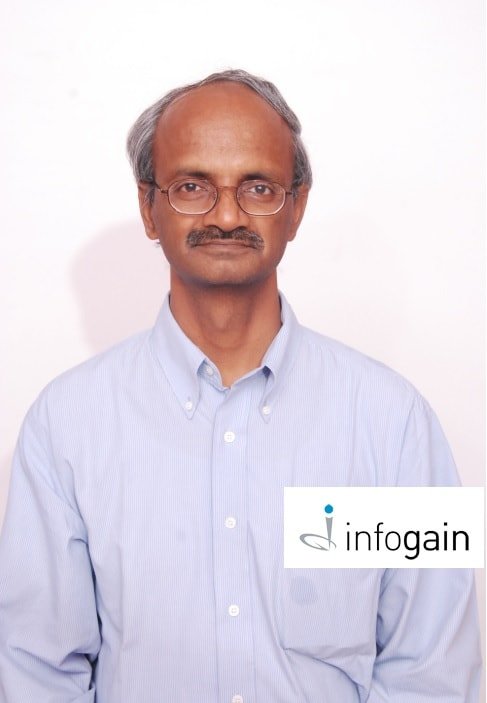
In the world of fast changing technology, Artificial Intelligence (AI) and Machine Learning (ML) are the front runners in creating the next disruption. The technology team at Infogain is at the forefront of understanding the potential of these new disruptive technologies. Team Estrade had the opportunity to speak to the man at the helm, Ramesh Subramanian, Chief Technology Officer, Infogain. Following is an excerpt of this interaction.
About Ramesh Subramanian
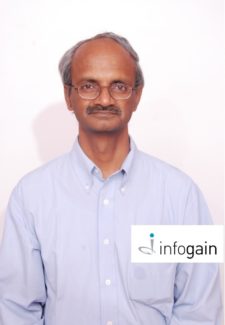
Ramesh is the Chief Technology Officer for Infogain. With over 30 years’ experience in multiple roles at global IT and consulting firms, his expertise spans business strategy, process improvement, global delivery, and pre-sales management. Prior to joining Infogain, Ramesh was the Global Delivery Head for Blue Star Infotech Ltd. His responsibilities included ensuring excellence in delivery, deploying solutions, and maintaining customer relationships. Over the years, Ramesh has served in various leadership roles including CEO, Vice President- Services, Director, practice head, sales lead, management consultant, and business analyst for IT and consulting companies that include KPIT Technologies, Tata Unisys, Accenture and Capgemini. Ramesh is an alumnus of the Indian Institute of Technology (IIT), Mumbai and the Indian Institute of Management (IIM), Bangalore.
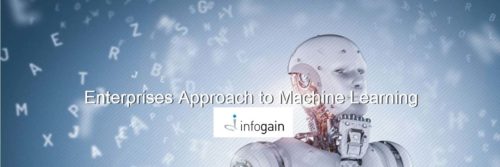
About Infogain
Infogain is a leading business and IT consulting firm specializing in business process, architecture design, end-to-end project implementation, and managed services. With more than 1,300 employees, Infogain has built its industry-specific business consulting and technology solutions to deliver value and competitive advantage to leaders in the Retail, High Tech and Insurance industries. Headquartered in Silicon Valley, CA, Infogain has additional offices in Irvine, Austin, Atlanta, United Kingdom, India, and the Middle East.
- How are enterprises today adopting Machine Learning and Artificial Intelligence?
Machine Learning and AI have advanced significantly in the past few years and enterprises are constantly working on models that would help in analysing large volumes of structured and unstructured data types with the ultimate goal to improve business performance. According to a latest Gartner prediction Artificial intelligence will be the top investment priority for 30% CIOs by 2020 as AI technologies will be pervasive in almost every new software product and service.Effective adoption is still in the nascent stage though, with evolving problem definitions, drivers, data sets and ROI expectations. ML is at a similar point on its adoption curve as the internet was in the late 1990s or cloud computing was in the mid-2000s. These disruptive technologies hold tremendous potential but the effort required is of a very different order from what commercial enterprises are used to, so enterprises need to step with caution and should have a well-thought out, strategic approach before adopting it.
“Early ML/AI pilots are unlikely to produce the dramatic results that technology enthusiasts predict.”
- Enterprises has seen tremendous changes in the last few years from adoption SaaS to Mobile and Analytics. How do you foresee the market changing with automation and intelligence?
The adoption of SaaS, Mobility and Analytics are a part of the trend towards “personalization”-of-business models driven by IT, where segments-of-one are the goal and the power of search and selection at the hands of the customer are practically infinite. Automation and Intelligence are intuitively the next steps in this trend, and we foresee these techniques becoming ubiquitous in the next year or two.
We are also witnessing the rise of conversational commerce, which emphasises speech and “intuitive” modes of interaction with service providers- human or otherwise; eg. Alexa-based amazon services.Such modes of interaction are forecast to replace Mobile Apps in the medium term (over 5 years). While inevitable, these modes are not robust as of date, and much effort remains in establishing them in the commercial environment.
Today, Machine Learning and AI is heralding new algorithms, applications, and frameworks bringing greater predictive accuracy and value to enterprise data. With the availability of huge data sets and the low cost of computing and storage, enterprises now are keen on exploring new technologies that can redefine their processes, bring in innovations and helps them to stay ahead of the curve. Automation is being implemented across industries, ranging from transportation and utilities and manufacturing and different sectors have their own sets of business benefits.Different industries will have to implement and apply automation in different ways to achieve the desired results.Some use cases for Automation and Intelligence transcend industry verticals- such as Chatbots for assisting with customer/ employee interactions, Advanced Search for better leverage of knowledge capital, Robotic Process Automation to integrate and streamline business processes; in more detail,for a Retail business, automation and intelligence can help in Predictive Inventory Planning, for a Travel business it can streamline traffic patterns and congestion management. Similarly for Healthcare it can help in real time patient monitoring and prognosis, and for a Manufacturing business, it can help in predictive maintenance and improved collaborative planning.
“Some use cases may require much more investment than others to “prove” the value-at-scale.”
- What kind of caution or preparedness is required by enterprises to adopt these technologies?
Enterprises are bound to face some challenges with Automation and Intelligence. At the highest level are the well-known risks to jobs arising out of more decisions being made automatically by machines, and improved digitization from process automation.
On the other hand, evidence suggests that early ML/AI pilots are unlikely to produce the dramatic results that technology enthusiasts predict. For example, early efforts of companies developing chatbots for Facebook’s Messenger platform saw 70% failure rates in handling user requests. Very few ML/ AI use cases move out of the labs into regular commercial usage even today. Firms need to understand that this technology represents a completely different paradigm and requires a well-thought out, strategic approach to adopting it.
The following steps/ approach can be leveraged to effectively operationalise enterprise ML/AI programs-
- Prepare groundwork for adoption– Machine learning based use-cases cannot be implemented overnight. Preparation for leveraging ML and AI technologies must be planned just as well as implementation of the technology itself. The business challenge needs to be articulated clearly and data required must be identified, prepared and manged on an ongoing basis. Identify the features required and engineer the architecture for building the same.
- Build Required Capabilities- Hiring the required skills is essential for deploying sophisticated ML and AI programs. This is probably the biggest challenge today, given the high demand for such skills but severe shortage in supply. Skills that are essential for ML include- modern architecture and technology skills, data science and experienced domain skills to understand complexity and requirements of implementing an industry use- cases
- Plan for Deployment at scale- Too many ML/AI projects fail today when implemented at scale, even though they might have had a successful PoC. Hence the planning for deployment must take into the account the scale for implementation- it should be well defined mathematically and the ontology that is developed must be robust at scale. The algorithms need to be trained adequately by ensuring availability of large and clean data sets
“We have helped our customers to make quicker and better decisions based on image analysis and monitoring”
- What kind of investments are required by enterprises?
Tech giants like Apple, Amazon or Google are investing in billions in R&D and in acquiring AI based start-ups. Typical investments required by all enterprises include the usual suspects: people, tools, time.
Some use cases may require much more investment than others to “prove” the value-at-scale. For example, to create a standard Chatbot supported by AI, an enterprise can go to the extent of hiring knowledge managers, data scientists, technology professionals that work with AI tools, even script writers of the kind used by call centers to handle input into the Chatbot. Besides these, of course, are investments in hardware, isolated environments and Big-Data storage.
The investment in people is, usually, the biggest cost- and these are people with high motivations and low tolerance for usual corporate bureaucracies; hence the tactic of “skunk works” is adopted by many enterprises, which adds to investment budgets.
- How is Infogain helping enterprises? What kind of solutions are you bringing to the market?
Infogain is at the forefront of implementing some aspects of the Automation and Intelligence technology spectrum. We help our customers automate and digitize business processes across countries and business groups, resulting in visible and significant benefits to their bottom lines.
We help our customers get a perspective on value of such technology to their businesses, identification of use-cases within their range of activities, and implementing pilots to review outcomes and caveats. This phase of ‘digital’ consulting enables our customers to plan strategically and adopt these technologies to the extent and within constraints as relevant to their businesses. While this still gives some exemplary results to our customers, such a methodical approach allows them to approach the business case without too many surprises.
We have helped our customers to make quicker and better decisions based on image analysis and monitoring- including video monitoring, in an automated manner; these customers span industries as wide as Finance, Insurance, Healthcare, Manufacturing, Travel, etc. Some of these use cases involve a combination of cutting-edge technologies, such as IoT, remote sensing and imagery, blockchains etc. alongside Automation and Intelligence to deliver value that was impossible in an earlier era.
We also actively induct partners and alliances into our portfolio, to ensure the entrepreneurial technology initiatives are made fully available to our customers.
“Machine Learning & AI heralding new algorithms” – Ramesh Subramanian, Infogain
Machine Learning and AI is heralding new algorithms